Database Centre
This blog is to share and exchange information related to databases
Thursday, July 14, 2011
SQL Server 2008 Performance Data Collector
SQL Server 2008 introduced Performance Data collector to overcome some of these limitations. Here we will see what exactly performance Data collector is and what it does.
Performance Data Collector is performance tools introduced in SQL Server 2008. It can collect data from single or multiple SQL Server instances and diagnostically analyze this information to generate reports. The activities of Performance Data Collected can be broadly classified into three categories.
Collects Data:
SQL Server 2008 comes with three built-in Data Collection Sets: one is to collect Disk Usage information; another is used to collect Query Statistics, and the third is used to collect a wide variety of Server Activities. According to Microsoft, these three Data Collection Sets collect the essential data needed to identify and troubleshoot most common SQL Server performance problems. If you don’t think the data that is collected is enough, you can create your own custom Data Collection Sets.
Central Repository:
All data collected by Data Collection Sets are stored in a centralized location called Management Data Warehouse (MDW). MDW can store data from single or multiple SQL Server Instances. While currently the focus of MDW is to store only performance related data, but in future version of SQL Server this will store virtually any data you want to collect from SQL Server, such as Extended Events, Audit data etc. The MDW is extensible, so if you are up to the task, you can store your own data in the MDW.
Performance Reports:
Data collected in MDW are diagnostically analyzed and reports are generated. SQL Server 2008 includes three built-in reports; Disk Usage Summary, Query Statistics History, and Server Activity History.
Friday, June 18, 2010
Sorting version numbers
CREATE TABLE version_list
(
ID NUMBER(10),
VERSION VARCHAR2(30)
);
INSERT INTO version_list VALUES
(1, ‘10.2.3’);
INSERT INTO version_list VALUES
(2, ‘9.1.2’);
INSERT INTO version_list VALUES
(3, ‘10.1.2’);
SELECT *
FROM version_list
ORDER BY version;
10.2.3
9.1.2
10.1.2
You can see that the output is not sorted.
SELECT *
FROM version_list
ORDER BY TO_NUMBER(REPLACE(version, '', ''));
9.1.2
10.1.2
10.2.3
Now you can see that the output is sorted. We had strip all dots from the version_list and then issued ORDER BY and it worked.
Sunday, June 13, 2010
Introduction to Data warehousing
In this article we will learn what is a Data warehouse? Why do we need a data warehouse? What are the characteristics of a Data warehouse? And finally go through some of the popular approaches for designing a Data warehouse.
What is Data Warehousing?
Ralph Kimball states that a data warehouse is a “Copy of transaction data specifically structured for query and analysis”
In other words; data is extracted periodically from production databases and copied onto special repository database. There it is validated, reformatted, reorganized, summarized, restructured, and merged with data from other sources. The resulted database is used to generate various summarized and ad-hoc reports, which in turn help in identifying future trends and decision making.
Why do we need a Data warehouse?
In today’s challenging times and cut-throat competition, there is a need to identify current trends, predict future trends and takes appropriate decision accordingly. Good decision can be taken only when we have all the relevant data available. Here the need of a good Data warehouse comes. A Data warehouse collects data from disparate sources and integrates them into a single source. Analysis is done using this centralized source.
Advantages and Disadvantages of a Data warehouse
Advantages:
- Large quantify of information consolidate into one location from disparate sources is available for analysis.
- Data warehouses facilitate decision support system applications such as trend reports (e.g., the items with the most sales in a particular area within the last two years), exception reports, and reports that show actual performance versus goals.
- Prior to loading data into data warehouse all data inconsistencies are identified and removed. This greatly simplifies reporting and analysis.
- Since Data warehouse is separate from operational system, they do not affect or slow down the operational system.
Disadvantages:
- Data warehouse system is complex in nature and they require skilled technical peoples and project managers.
- Setting up a Data warehouse is very expensive and time consuming.
Characteristics of a Data warehouse:
According to Bill Inmon "A warehouse is a subject-oriented, integrated, time-variant and non-volatile collection of data in support of management's decision making process"
Characteristics of a Data warehouse are explained below:
Subject-oriented: Information is present based on specific subject. Data is organized in such a way that data related to all real word elements are linked together. E.g. in operational database data will be grouped based on Loans, Savings, Demat etc. where as in warehouse data will be grouped based on subject like Customer, Vendor, Product etc.
Non-volatile: Data once entered is never updated or deleted. It is remains static and read-only for future reference.
Integrated: Data warehouse contains data from different operational data sources (OLTP) into a single integrated database.
Time Variant: Data warehouses are time variant in the sense that they maintain both historical and (nearly) current data.
Accessible: The primary purpose of a data warehouse is to provide readily available information to the en-users.
Implementation Methods
These are broadly two main approaches for designing a warehouse.
Top-down Approach
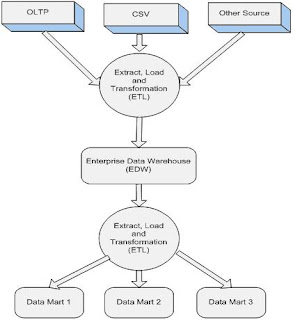
This is a Bill Inmon’s approach. In this approach data is extracted from operational system and loaded into staging area. Here the data is cleansed, consolidated and validated to ensure its accuracy and then transferred to Enterprise Data warehouse (EDW). The Data in EDW is usually in normalized form to avoid redundancy and have a detailed and true version of data. After EDW is in place, subject area specific data-marts are created which have data in de-normalized form and in a summary format.
Top-down approach should be used when:
We have complete clarity of Business requirements related to all verticals.
We are ready to invest considerable time and money.
Bottom-up Approach:
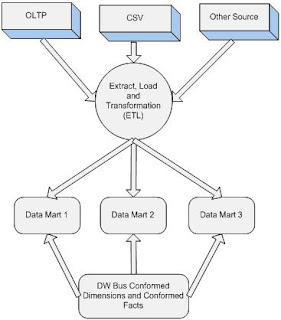
This is a Ralph Kimball’s approach. In this approach data marts are build first and then gradually the final Enterprise Data Warehouse (EDW) is build by integrating different data marts. Here separate data marts are combined based on Conformed dimensions and Conformed Facts. A Conformed dimensions and Conformed Facts can be shared across data marts.
Bottom-up approach should be used when:
We have time and money constraint.
We do not complete clarity of all verticals of Business and have clarity of only one or two business vertical.
Data warehousing Terms
Fact Table: A Fact table consists of the measurements, metrics or facts of a business process. It is often located at the centre of a star schema or a snowflake schema, surrounded by dimension tables. E.g. In a banking data warehouse accounting and balances will be stored in a Fact table.
Dimension Table: A dimension table consists of textual representation of the business process. E.g. In a banking data warehouse accounting and balances will be stored in a Fact table where as customer name, address, mobile numbers will be stored in the dimension table.
Cube: Cube is a logical schema which contains facts and dimensions
Star Schema: Star Schema is simplest data warehouse schema which consists of a few fact tables and multiple dimension tables connected to fact table.
Snowflake Schema: Snowflake schema is a variation of Star schema where dimension table are normalized into multiple related tables. Fact table remains same similar to Star schema.
Data Mart: Data marts are analytical data stores designed to focus on specific business functions for a specific community within an organization. A single Data warehouse may have multiple data marts.
Extract Transform and Load (ETL): It is a process through which data is extracted from disparate sources, transformed as per the requirement and loaded to the target database.
Operational Data Store (ODS): It comes between Staging Area and Data warehouse. Data is extracted from OLTP system to stating area and then after cleansing and validation, data is loaded into ODS. Later on data is summarized and loaded into Data warehouse.
Data Mining: Data mining (sometimes called data or knowledge discovery) is the process of analyzing data from different perspectives and summarizing it into useful information - information that can be used to increase revenue, cuts costs, or both.
Business Intelligence (BI): Business intelligence (BI) is a broad category of applications and technologies for gathering, storing, analyzing, and providing access to data to help enterprise users make better business decisions. It involves data mining, business performance management, benchmarking, text mining, and predictive analytics
Conclusion
We had learn that in Data warehousing data is collected from different sources, cleansed, validated, summarized and stored in a separate repository called data warehouse. This data is then analyzed from different perspective in order to take good business decisions.
Monday, July 28, 2008
Sorting: Character should come first
SELECT id FROM test ORDER BY id;
ID
---
1
2
3
A
B
C
There could be case when we want character data to come first and then numeric data. This can be achieved by simple manipulation of ORDER BY clause as follows:
SELECT id FROM test
ORDER BY DECODE(REGEXP_REPLACE(id, ‘\d+’), null, 1, 0);
ID
---
A
B
C
1
2
3
REGEXP_REPLACE is a regular expression function introduced in Oracle 10g. Here by using this function we are identifying all numeric data and replacing it with NULL. After that using DECODE function all NULLs are replaced with 1 and the remaining character data is replaced with 0. Hay this is only done in ORDER BY field NOT IN SELECT. Now we can ensure that zero (character data) obviously comes before 1 (numeric data in our case).
Thursday, July 3, 2008
Loading data into Oracle from Text File
SQLLOADER is a bulk loader utility used to move data from external files into oracle database. Simple example of loading external file is given below.
First you need to create a control file which should look similar to the below example
CONTROL FILE:
OPTIONS ( SKIP=1)
LOAD DATA
INFILE 'C:\TextFile\emp.csv'
BADFILE 'C:\TextFile\emp.bad'
DISCARDFILE 'C:\TextFile\emp.dsc'
INTO TABLE "SCOTT"."EMP"
INSERT FIELDS TERMINATED BY ','
OPTIONALLY ENCLOSED BY '"'TRAILING NULLCOLS
(
EMP_ID sequence(Max, 1),
EMP_NAME,
EMP_DEPT,
EMP_ADDRESS,
EMP_DOB
)
If your .csv file has headers then you need to add SKIP = 1.
You need to make sure that you have emp.csv exist in the given location. Other files like .bad and .dsc will be generated by sqlloader itself, if required.
Now save this control file on any directory (eg. c:\LoadTest\Emp.ctl)
To execute this, Type following command in the command prompt
c:\sqlldr userid=scott/tiger@orcl control=c:\LoadTest\Emp.ctl log=c:\LoadTest\Emp.log
Copying table from one database instance to another without using dblink
Querying current value of sequence without incrementing it
Eg.
CREATE SEQUENCE seq1
START WITH 1
INCREMENT BY 1
CACHE 20;
If we try to issue SELECT seq1.CurrVal FROM dual without issuing se1.NextVal. It will give error stating that sequence is not yet defined in this session. But, if we use seq1.NextVal, it will increment sequence by 1 which we don’t want. So here is one easy way for getting this:
ALTER SEQUENCE seq1 NOCACHE;
SELECT last_number FROM user_sequences WHERE sequence_name = ‘seq1’;
ALTER SEQUENCE seq1 CACHE 20; --* or whatever is defined earlier